The medical jump from mice to humans just got easier
Machine learning technology could help researchers determine what mouse data are useful when designing human clinical trials
Rama on Wikimedia Commons
Scientists have made thousands of breakthroughs studying diseases that plague the human population. But there’s one major problem: Most of those discoveries were made in mice, and more often than not, those discoveries don't translate to humans.
Now, a team at the Technion-Israel Institute of Technology has used machine learning to develop a computer model that predicts which medical breakthroughs discovered in mice might hold true in humans. If the technology pans out, it could save time and money otherwise spent on clinical trials and shorten the path to treatments for diseases like Huntington’s and cystic fibrosis.
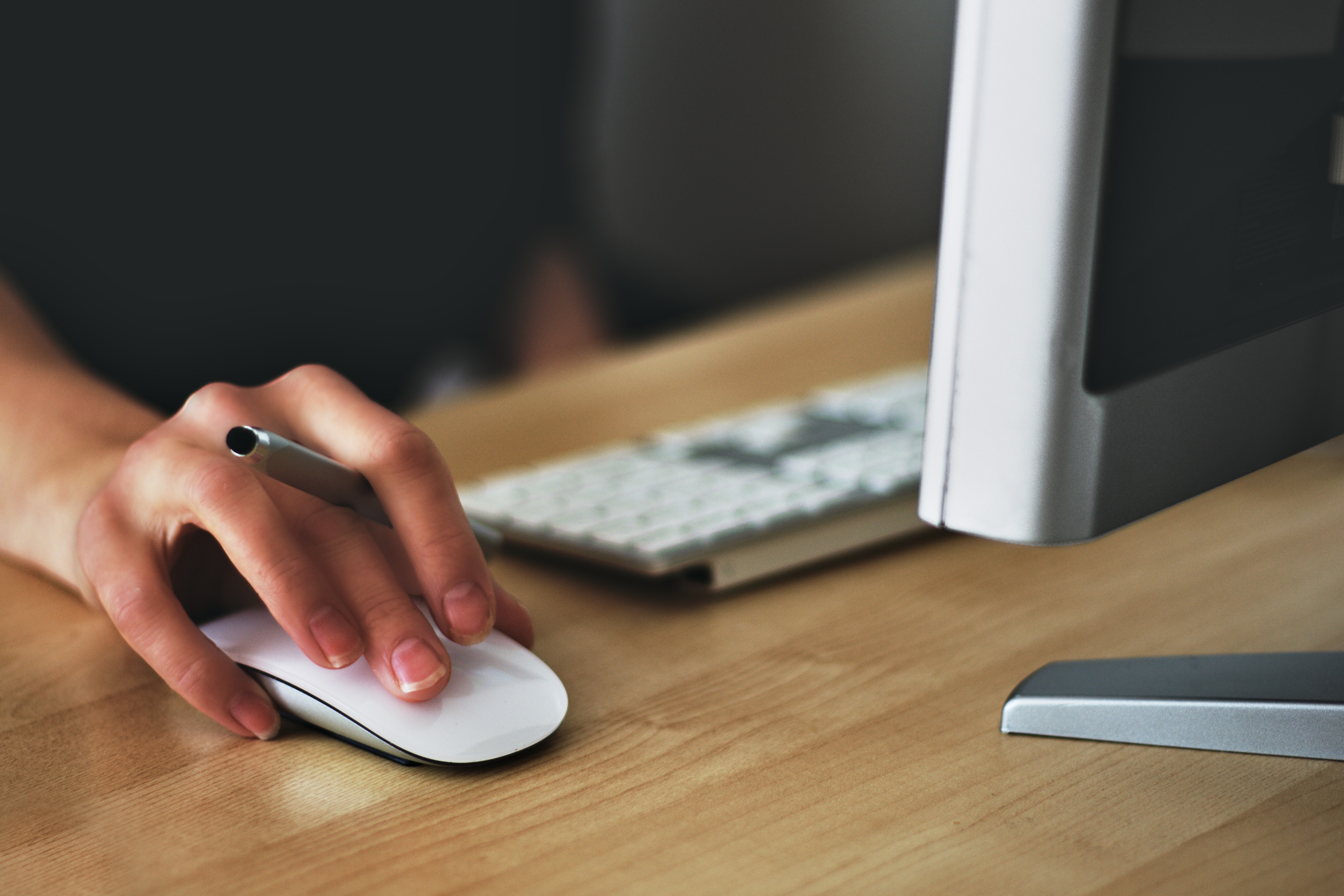
Scientists are hoping to rely more on this type of mouse than the traditional lab mouse in designing future clinical trials
Photo by Vojtech Okenka from Pexels
Mice are the go-to animal for testing the efficacy of new drugs and therapies. They share 78.5% of their genes with humans, and are engineered by scientists to share many of the same diseases. The shorter reproductive cycles and lifespans of mice enable scientists to collect data in weeks or months instead of years. And experimenting on mice comes at a fraction of the cost of human studies without the strict ethical constraints.
Despite these similarities, “mice and humans [have] evolved to be quite different,” says Robert Perlman, Professor Emeritus in the Department of Pharmacological and Physiological Sciences at the University of Chicago and author of the book Evolution and Medicine, who was not involved in creating the model. “It’s not as though studying mice [is] bad,” he adds, but “many studies in mice can’t be translated to humans.”
One immune stimulant drug study, for example, showed promise in mice and primates, but led to multiple organ failure in six healthy men. In the 1950's, the drug Thalidomide was prescribed to pregnant women after it was shown to successfully alleviate morning sickness in mice. The drug ended up causing birth defects that led to thousands of deaths.
Harmful human trials aren’t the only potential downside to relying on mouse research. With the United States spending billions of dollars on drug development each year and total clinical trials succeeding just 14% of the time, something needs to change.
“There will always be a need for mice in biomedical research, but we need to somehow do it better,” says Rachelly Normand, the computational biologist who led the development of the computer model, published in the journal Nature Methods.
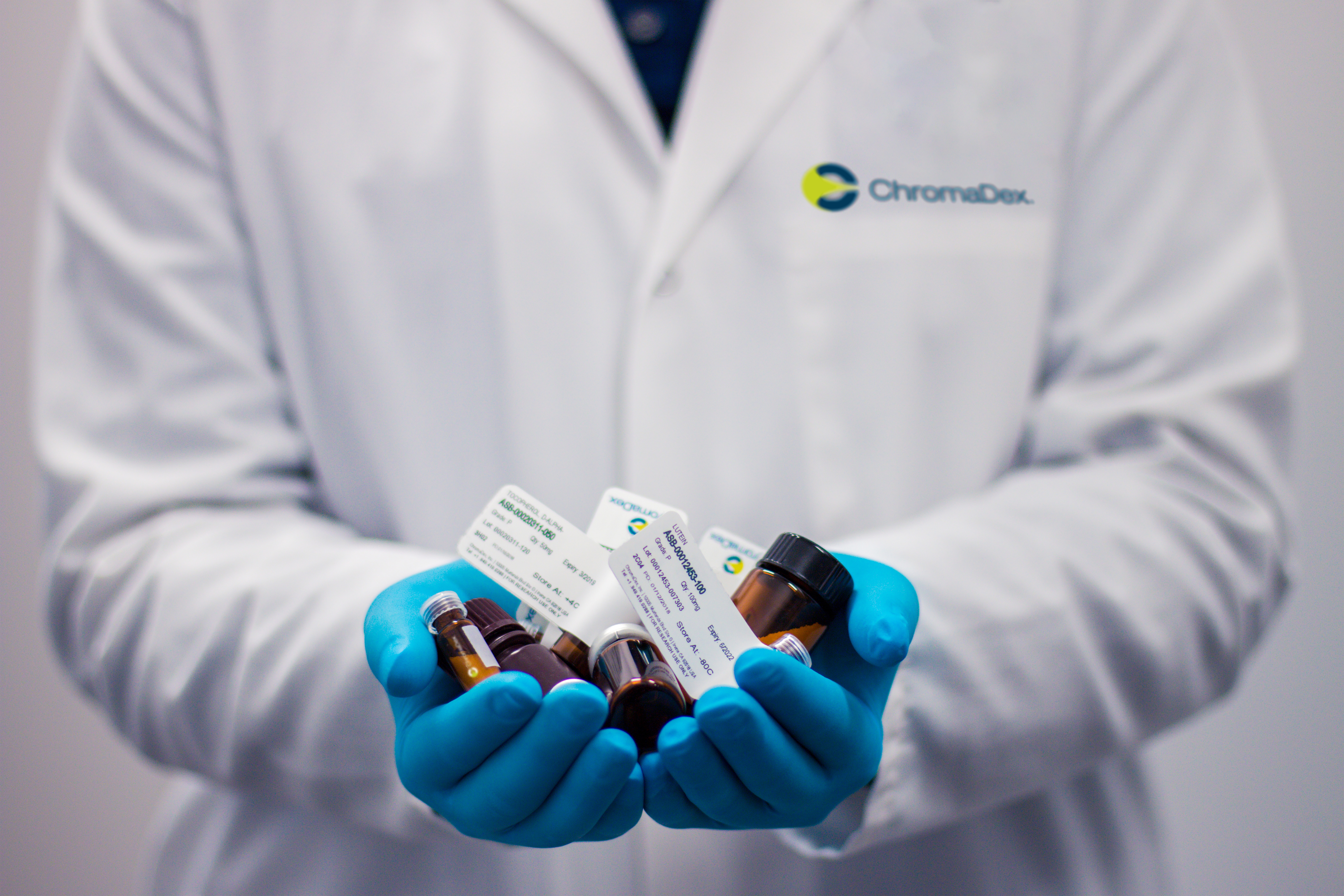
Data from lab mice does not always correlate with results from human clinical trials
Photo by Kendal James on Unsplash
This model, which Normand and her colleagues named Found in Translation, or FIT, determines which results from mouse studies will translate to humans. It works by predicting the likelihood that disease-associated genes in mice will be important in the equivalent human disease and how well drugs that target those genes will translate across species.
For example, a drug may cure diabetic mice by shutting down a mouse gene that's causing the disease. In people with diabetes, this same gene may behave differently, and the drug that worked on mice may not be useful – and may even be harmful – in people. Before testing the drug in humans, the mouse study results could be evaluated by FIT.
The researchers taught FIT to assess mouse studies by feeding it publicly available data from previous research looking at both mice and humans. This “training data,” as the scientists called it, was carefully vetted by the researchers and consisted of 170 studies that investigated the same disease-related genes in both species, as well as genes associated with health. The training dataset taught FIT which biological features mice and humans share — and which they don’t — so that it could make future translation predictions.
Perlman believes data vetting is the essential step in designing the model. “Machine learning is only as good as the quality of the data that are given [to the] machines.”
At the end of the training, the researchers were able to feed FIT data from a promising new mouse study. FIT spat out a number for each gene in the database. The higher the value, the more likely the mouse findings related to that gene would translate to humans.
To test the model’s accuracy, the scientists entered mouse data for genes that had already been studied in humans. Then, they compared FIT’s prediction to the already-collected human disease data.
The model also incorporated a pre-step, which determines if FIT will be helpful in the first place. This depends on if the model has encountered the relevant mouse genes in its training or not. This pre-step, or classifier step, first compares the new mouse data to the training dataset. If the two datasets are too dissimilar, FIT shouldn’t be used.
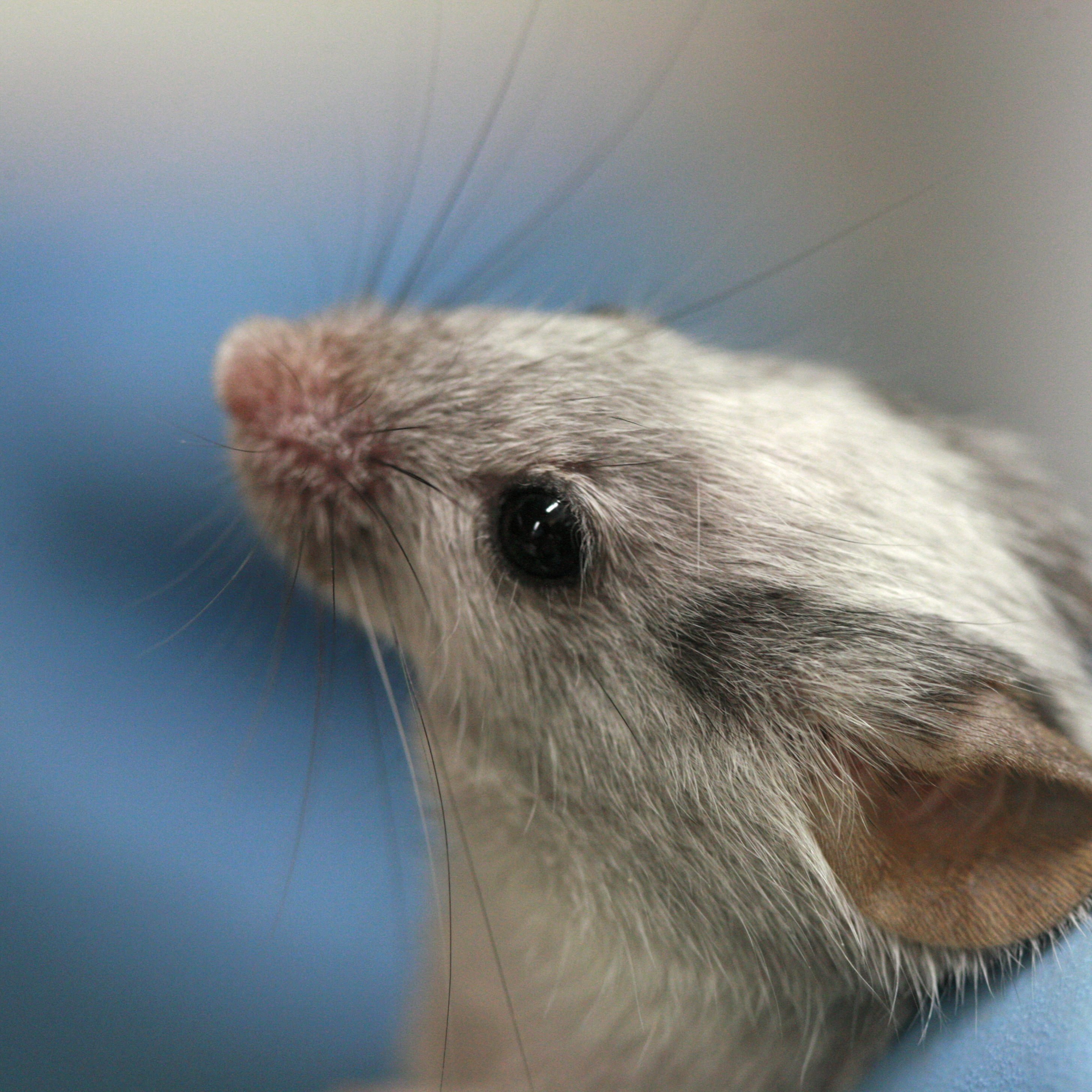
Rama via Wikimedia
About three out of four of the studies weren’t considered relevant during the classifier step, in which case FIT wouldn’t have been a helpful tool for telling if the mouse data would translate to humans, Normand and her colleagues say. But of the quarter of studies that were deemed to be eligible for FIT, its translation predictions were correct 88% of the time.
Normand hopes the model will be adopted widely among researchers to better design clinical studies. “Anyone without coding experience or computation experience should be able to use it pretty easily,” she says, citing FITs online availability and user-friendly format.
Mice, while vital to biomedical research, are still imperfect test animals for studying human diseases. Machine learning could help make mouse data more translatable, saving both dollars and lives.
Peer Commentary
Feedback and follow-up from other members of our community
Carina Seah
Stem Cell Biology
University of Southern California
What an interesting article! The idea of an algorithm to determine how well mouse studies might translate to human results is extremely thought-provoking. It is absolutely true that results in mice oftentimes do not translate to results in humans, and this predictor could help identify the specific diseases and mutations that would most benefit from using mouse models. I also appreciate that you mentioned that computer models are only as good as their data and that this algorithm could be improved upon with more genes studied in both mice and humans. However, as a neuroscientist, many times there are no better models out there. For example, a neuroscientist studying Alzheimer’s disease may be unable to study behavioral defects in cell culture and therefore rely on mouse models, even though there are not a lot of similarities in mouse behavior and human behavior. I am worried that in these cases, algorithms such as these would invalidate the use of mouse models when they may be the best model we have. Ultimately, though, I keep thinking about how cool this would be if we could apply it to other model organisms outside of mice. Where mice and humans may differ, perhaps another, less used model organism may actually have greater similarities. Imagine if researchers studying a gene could go into a program that let them know what model system would be the most accurate for their research?
I really enjoyed this article and I think the computer model shows great promise for translational research, but I agree with Carina. I struggle to see that we’re currently at a point where we can use computer modelling to predict complex systems such as the nervous system. What struck me about the article was how gene oriented the computer model is. I was left wondering whether the model would perform for translating treatments that aren’t strongly gene based but mechanism based or if it really is solely for gene-based research.
Madison Hansen
Computational Biology
American Museum of Natural History
Thanks so much for sharing this interesting article. I think the article does a great job of describing machine learning methods in a way that is not intimidating, it’s very accessible. I did have some questions while reading the article. Do researchers currently just test everything on mice? Or do they have an existing method for determining if testing in mice could translate to humans, and if so, what is that method? I also had questions about the research itself. How many genes were included in the FIT database, and what percent of all relevant mouse genes could FIT be applied to? How many studies per gene were fed to the model? 170 studies of two genes is a decent amount of data per gene. But 170 studies of 17 genes is only 10 studies per gene. I hope the researchers are planning to expand their database of studies so that it can be applied to more of the mouse genome and make better predictions.
Katherine Basil
Neuroscience
Maastricht University
This is a great step forward in lab animal studies, specially that the field has been facing several drawbacks in poor translation to the bedside. But I personally believe we will slowly move more into human organoid models which have been showing great promises in a variety of disciplines (including neuroscience!). We have invested a lot of time and money on rodent animal studies, which have certainly helped us learn A LOT about rodents and to a lesser extent about humans and human diseases. I like to always stress on the poor validity of these models when looking at psychiatric disorders. For instance, a sucrose test is NOT indicative of depressive symptoms that are typically seen in humans. Psychiatric disorders like depression are much more complex than what a mice or rat experience. Nevertheless, not to be the pessimistic one here, I am in favour of Carina's final suggestion/question: “Imagine if researchers studying a gene could go into a program that let them know what model system would be the most accurate for their research?” I think it is time we let go of what we previously thought was THE model system of all, and look into new fresh territories with great(er) promises.
Sophie Okolo
Health Technology
Very informative and insightful article! As machine learning technology continues to advance, I’m sure there will come a day - hopefully in the nearest future - when researchers can determine the most useful mouse data. I’m more curious about other potential animal models for gene-based research. Do they exist? Carina raised a point about the often lack of better models and why we may use machine learning to determine certain characteristics of models such as the potential impact of treatment. Perhaps new bioinformatics tools will create accurate and reliable mouse models that can translate to humans.