Math models can help cardiologists by finding the heart's hidden waves
Even seasoned physicians may struggle to interpret EKGs
Hermes Manuel Cortés Meza from Pixabay
Whether you call it an ECG, or an EKG — or even an "electro" as hip Chileans do — an electrocardiogram is a common screening test used to evaluate heart health. The abbreviation "EKG" comes from the German spelling of "elektrokardiogramm," and is often used to prevent confusion since ECG sounds like EEG (short for electroencephalogram), a completely different test that measures brain waves. Unfortunately, the naming scheme isn't even the most complicated part of the exam. Primarily used to diagnose problems like arrhythmia and coronary artery disease, the squiggly lines of EKG results can be challenging for doctors to interpret. But luckily, researchers have recently developed a new mathematical model to greatly improve the diagnostic accuracy of EKGs.
During the procedure, small plastic electrodes are attached to the skin on a person's chest, wrists, and ankles, and then used to measure the electrical activity of the heart. The process doesn't involve sending any electricity into the body, just detecting electrical pulses that generate the contractions of the heart muscle. This shows the speed at which the heart is beating, the rhythm and timing of different parts of a single beat, and the strength of the muscle contractions.
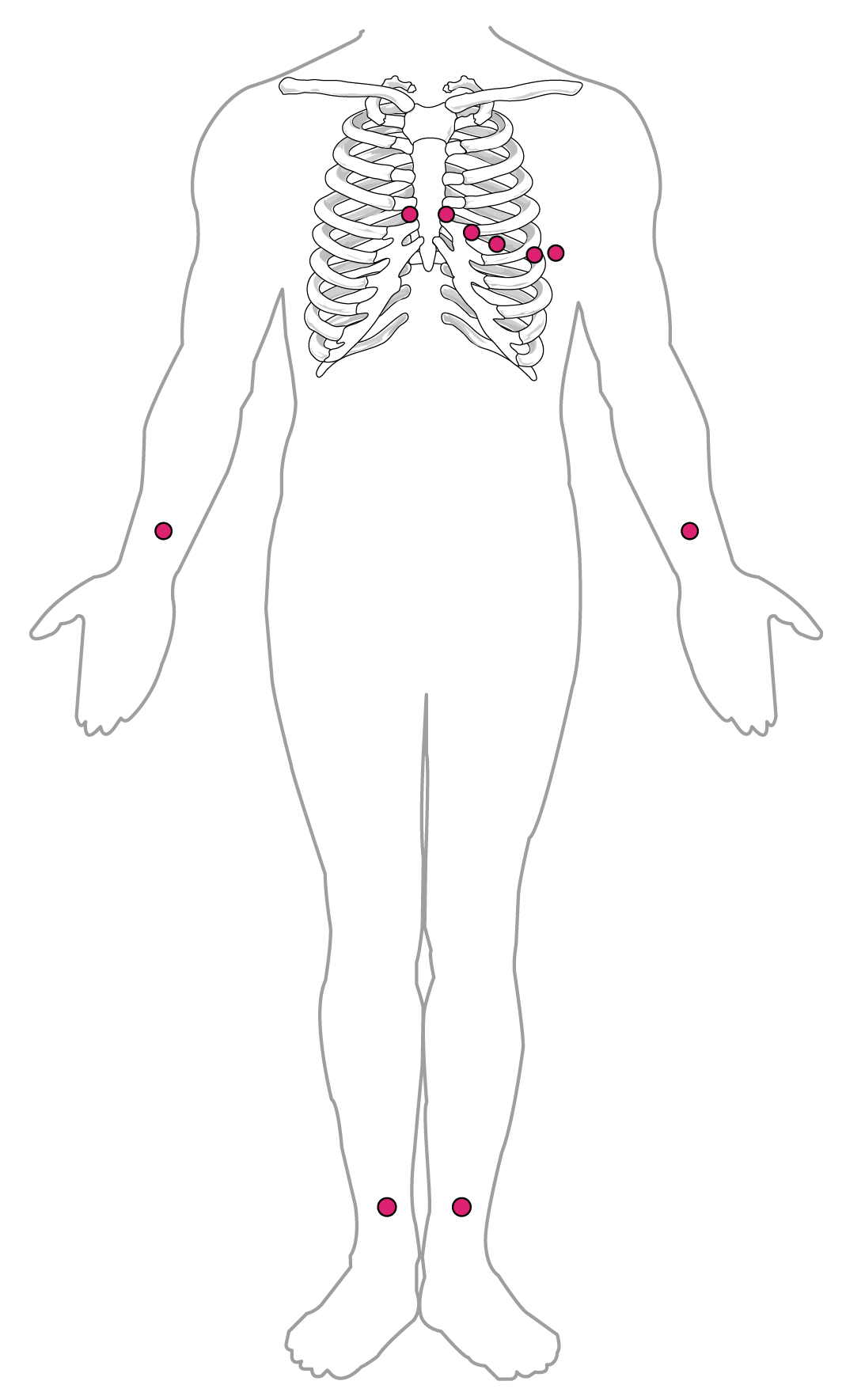
Standard placement of EKG electrodes.
OpenStax College, CC BY 3.0, via Wikimedia Commons
Electrocardiograms are mostly used as diagnostic tools, since an irregularity in any of those measurements could indicate a heart condition. However, interpreting sine-like squiggles on graph paper is not easy, even for trained physicians. There is a huge diversity of abnormalities that can appear, and the mapping of results to diagnoses is not always consistent. Different heart problems can generate very similar results, and a single condition may be associated with more than one abnormal pattern.
Learning to accurately read electrocardiograms requires memorization and practice, so turning to computers for help is a logical step. It's not even a recent development: automated interpretations of EKGs have existed for over half of a century. However, even after 60 years of development, computer diagnostic accuracy is limited, and physicians still need to review all test results by hand. This isn't a case where a passing grade is good enough. When errors occur, lives are at stake.
At this point in the technology's development, automated EKG results are meant to assist experts, not replace them, and researchers agree that manual verification is mandatory. Unfortunately, that assist isn't always helpful. Multiple studies have shown that physicians are influenced by an automated diagnosis, and troublingly, that they tend to agree with it, no matter whether it is right or wrong. In other words, if two physicians are shown the same squiggly lines, the one that sees a misdiagnosis from an automated system (without knowing it is wrong) before manually reviewing the results is more likely to misdiagnose the patient than a physician who interprets the EKG without knowing what the automated system predicted. This demonstrates the critical need for better automated analyses, as well as a better way to validate those analyses.
So far, there have been two main approaches to developing a better automated electrocardiogram interpretation tool: mathematical models and machine learning.
Various math-based models have proved accurate for specific heart conditions but aren’t useful as a general, multipurpose solution. These models tend to fail primarily for atypical EKGs — which is problematic as this test is primarily used as a diagnostic tool, and atypical results are the most important ones to decode.
In contrast, machine learning approaches for EKG interpretation can be used for a broad range of heart conditions, but they are highly dependent on what's included in the training dataset and don’t provide much information beyond the diagnosis itself. During development, the program must be trained on an existing collection of EKG results, which often do not include a complete collection of abnormal heart conditions, and thus introduce bias into the algorithm. Moreover, the black-box nature of machine learning solutions is particularly dubious because it gives physicians a diagnosis without any context, and without a way to efficiently verify the results.
Computer programs also face challenges that people don't. For example, EKGs with noisy signals are challenging for an automated system to parse, but are very common due to things like muscle contractions, patient movement, and interference from neighboring electrical devices. It's also harder for a computer program to account for factors such as the patient's age, gender, and medications.
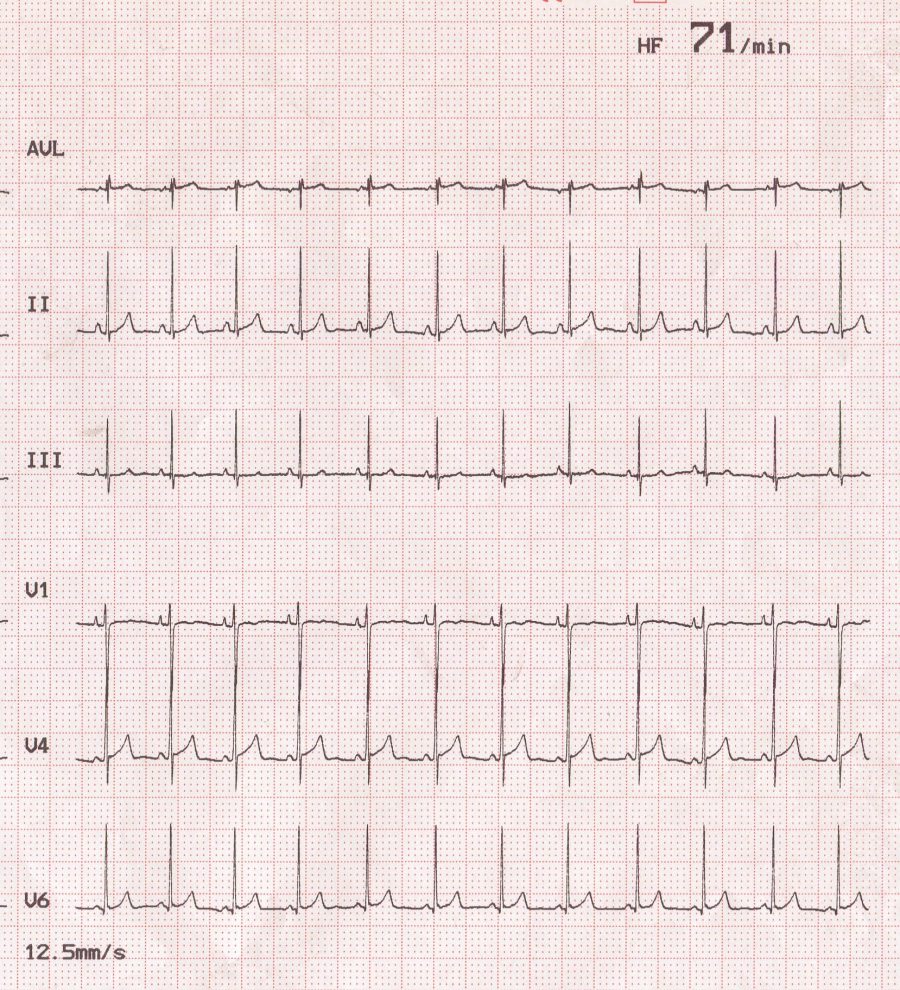
Normal EKG results.
Magdalena Wiklund via Flickr
To solve these problems, in early 2021 researchers published results detailing the development of a unique mathematical model to analyze an electrocardiogram. This new model uses some fancy math to separately characterize the five fundamental waves of a heartbeat, an approach that has proven to outperform the previous best wave detection algorithms. It does so by generating parameters describing the wave shape of a heartbeat, similar to what a physician would look for manually in the squiggles. Diagnostic results are then calculated automatically from that data.
The parameters generated by this new model are different from previous models because they actually have physical meaning, and instead of a black-box system, they give real insight into how the diagnosis was made. They also do not change significantly with a noisy signal, an important advantage when considering imperfect data and older machines. To account for factors like age, gender, existing conditions, and medications, the parameters can be easily compared with reference values. This model is useful for patients of all ages and backgrounds, and is free from the race- and gender-based biases that often appear in artificial intelligence programs.
Manually verifying automated EKG results is still necessary, so this new approach saves time by quickly generating measurements that a physician would normally have to do by hand. Then, they can use that information to more efficiently double-check the diagnosis.
However, it's not quite ready for the emergency room. There are a few heart irregularities that do not fit in well with the new model, since some conditions, such as an atrial flutter, do not always produce a recognizable, repeated wave pattern. This can be addressed in the future by creating a specific algorithm for these cases to use in conjunction with the model. The researchers also note that while their analysis tool performs better than previous models, its reliability could still be improved.
Their next step is to create a large database capturing outliers and develop specific algorithms for conditions that aren't well-defined by the five main wave types of a typical heartbeat's electrical signal. This will improve the range and reliability of the model. The complete analysis tool will need to be fully tested by researchers, and then approved by the U.S. Food and Drug Administration before it can be used in the field.
It's a long road from published research paper to hospital use, but it's worth the effort to bring efficiency and reliability to medical clinics, especially in areas with fewer trained experts. Continuing with this type of research is important because it's another step toward lowering the barriers to quality medical care. While a trained physician is irreplaceable, this new user-friendly electrocardiogram interpretation program could help save lives.